January 17, 2022

Editors: Fabio Gomes, Medical Oncologist at Christie NHS Foundation Trust, Manchester, United Kingdom and Janine Capobiango Martins, Medical Oncologist at Prevent Senior, São Paulo, Brazil.
Older adults are underrepresented in cancer clinical trials1,2. Though some are excluded due to having more comorbidities than their younger counterparts, others decline participation because of an already high healthcare burden. Many older adults have limited mobility and/or rely on others for transportation, so each additional appointment is burdensome. This complexity is a disincentive for clinical trial enrollment and leads to a lack of generalizability for how to apply the newest treatments to a rapidly aging cancer population. One way to safely and easily involve older adults in clinical trials is to utilize new technologies that bring aspects of the trial to their homes through the use of wearable sensors and at-home biospecimen collection kits.
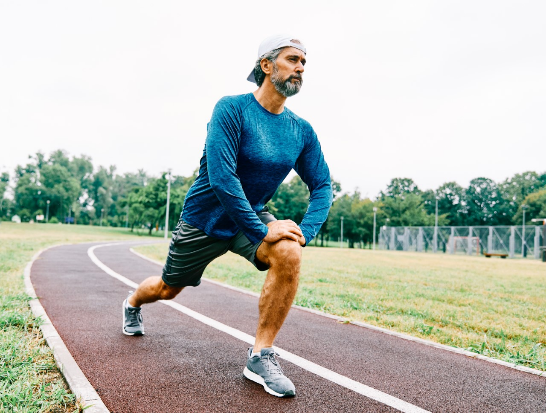
Wearable sensors, e.g. wrist-based activity trackers, glucometers, etc., are popular, diverse, and can provide many types of data that are relevant for clinical trials. The data collected are dense and longitudinal, enabling analyses that are impossible with other data types (Figure 1). We have recently adapted an algorithm that can be applied to mobile health applications, and created a tool to help detect whether or not an intervention has occurred for an individual3.
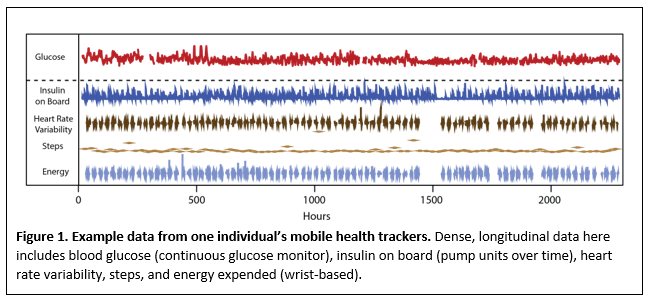
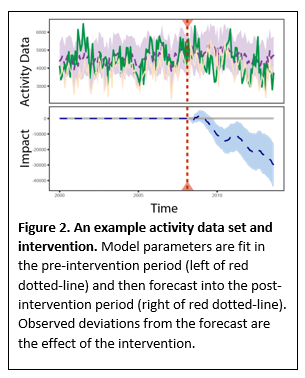
This uses the individual’s pre-intervention data (e.g. baseline data gathered a week before starting a physical activity intervention) to fit a set of model parameters and then forecasts their activity post-intervention. The difference between the observed and forecast data is the effect of the intervention (Figure 2).
These data can be wirelessly transmitted to healthcare providers to assess activity levels, monitor gait, sleep duration and quality, and/or measure blood glucose levels. In addition, pairing mobile health sensors with other biospecimen collections, such as at-home gut microbiome samples, compounds the value of the data. Gut microbiome and some cytokine information can be collected at home and mailed at room temperature to processing facilities, thus saving patients’ time and travel stress.
The collection of lifestyle data and its management is particularly important in the era of cancer immunotherapy treatment. The re-engineering of the body’s immune system by immune checkpoint blockade casts into sharp relief factors that affect immune system function, including diet, physical activity, and sleep. Older adults are at risk of being deficient in one or more of these areas, and this represents an actionable target to improve treatment outcomes. The collection of these data need not be burdensome to be beneficial or it does not need to include extra trips to the clinic but can illuminate aspects of health not currently explored.
Of course, there is no substitute for face-to-face interactions for assessing patients’ well-being, particularly for initial assessments. However, for subsequent visits, such as during cancer treatment on clinical trials, wearables and at-home kits are a safe and easy way to monitor patients while reducing the treatment burden on them and their caregivers. We hope this design will encourage more participation from older adults in clinical trials, and better inform our continued care of this growing population.
References
- Herrera AP, Snipes SA, King DW, Torres-Vigil I, Goldberg DS, Weinberg AD. Disparate inclusion of older adults in clinical trials: priorities and opportunities for policy and practice change. American journal of public health. American Public Health Association; 2010;100(S1):S105–S112.
- Sedrak MS, Freedman RA, Cohen HJ, Muss HB, Jatoi A, Klepin HD, Wildes TM, Le-Rademacher JG, Kimmick GG, Tew WP. Older adult participation in cancer clinical trials: A systematic review of barriers and interventions. CA: A Cancer Journal for Clinicians. Wiley Online Library; 2021;71(1):78–92.
- Liu J, Spakowicz DJ, Ash GI, Hoyd R, Ahluwalia R, Zhang A, Lou S, Lee D, Zhang J, Presley C, Greene A, Stults-Kolehmainen M, Nally LM, Baker JS, Fucito LM, Weinzimer SA, Papachristos AV, Gerstein M. Bayesian structural time series for biomedical sensor data: A flexible modeling framework for evaluating interventions. PLOS Computational Biology. Public Library of Science; 2021 Aug 23;17(8):e1009303.
- JAMA. 2012. November 21;308(19):1972–3. 10.1001/jama.2012.14541
Leave A Comment
You must be logged in to post a comment.